"Instead of looking back in anger or fear where we are wrong or how a bit of courage could have altered the course of events, we should look around with awareness to ensure our past actions don't become a habit" - Dr Prem Jagyashi
Sometimes it is required to write dispassionately and analytically about things that are controversial. I wrote this years ago and have returned to it multiple times since. COMPAS was a piece of machine learning that allegedly became racist and biased though this was not upheld by the supreme court. Over the course of this article I have tried to look at COMPAS as a retrospective and to more fully analyse what it means for organisations.
For me COMPAS is the anti pattern or otherwise what data science should not be and it has for years played on my mind as I returned to it multiple times mouthing the words how did they manage to do this. In doing this I have settled my mind on the history and even come to understand the defence and how those who supported COMPAS might phrase a reasonable counter argument. Having looked more deeply and reviewed modern approaches to data science projects in hope it would not be used today.
Description
COMPAS, an acronym for Correctional Offender Management Profiling for Alternative Sanctions. It was a piece of machine learning that was used to decide a defendant term of imprisonment following a guilty verdict by a jury. COMPAS has been used by the U.S. states of New York, Wisconsin, California, Florida's, Boward County and other jurisdictions.
COMPAS was developed by Northpointe there own description of the algorithm is found here FAQ_Document.pdf (northpointeinc.com).
COMPAS was designed so a Judge at trial could ask 137 survey like questions and receive a score that estimated the likelihood to reoffend. While race was not reported to be implicitly included in the data it is thought that the piece of machine learning may have learned race by using postcode as a proxy given the propensity of American cities to be racially segregated. Though even postcode does not appear to be a documented parameter on the companies own documentation; Therefore it is unclear what data was added or if the algorithm in actual use complied with Northpointe's own publications to create the situation that then followed.
A sample COMPAS report can be found here Sample-COMPAS-Risk-Assessment-COMPAS-"CORE" - DocumentCloud.
Questions used where: based on observations is the person a suspected gang member, How old where the individual when parents where separated. Amount of friends arrested, number of times moved during childhood, and postcode is used from residential and neighbourhood stability (a point by which it is assumed bias entered the system).
The model also used the defendants self admission to there mental state using anger, sadness and boredom. Therefore it is reliant on the defendant admitting honestly mental state. Finally it asks a series of ethics question like does a hungry person have a right to steal. All this together was meant to give a score of likelihood of a reoffence within 2 years.
In 2016 ProPublica published research that suggested that blacks where tice as likely than whites to be wrongly predicted as at risk of recidivism and the opposite is true for white people that it would predict no risk of recidivism but half would go onto recommit crimes. They also found out that only 20% off those predicted to reoffend did so.
ProPublica supplied data that they used to come to this conclusion that included postcode and race as labels for the individual offenders. I supply my own analysis below of this data. ProPublica did this analysis using data from 10,000 Florida defendants. A summary of ProPublica work can be found here How We Analyzed the COMPAS Recidivism Algorithm — ProPublica.
The subsequent study found that Compas was 65% accurate in getting the right answer. This was against a reported accuracy of 63% for random people and 67% for groups of people discussing there opinions and biases (i.e. juries). Oddly that appears to have been meant as a rebuttal of ProPublica's research. I note that Compas was not compared against professional law enforcement agents, lawyers and judges who will have already analysed the risk. It is also strangely not a rebuke of the statement that Compass is biased based on race.
A lengthy media and academic debate ensued. COMPAS was widely reported upon and the reason why many people choose to do case studies is the wealth of information available. Examples such as New Scientist report here Discriminating algorithms: 5 times AI showed prejudice | New Scientist.
It has been suggested COMPAS used social economic factors and that bias against race within policing caused other races to be assessed as having much lower social economic factors than they did and white people much higher. This was compounded with errors in police databases meaning that on retrieval a persons data was often incorrectly matched with other people.
This led to widespread reporting within the media as the algorithm being a demonstration as proof of bias within the police against different races. This appears a clear possibility as a number of the inputs where decided by the police and it was concluded that human bias might be the cause in that officers may statistically assess the social economic statuses as lower. Though the likelihood that indicators of race where included by proxy due to how racial groups congregated in American cities also seems plausible so it is not required that the police themselves be biased. This explanation was seized upon by the media reporting but not something that could be proven upon review of the authorities sources.
When looking at bias there appears two extremes of approach; just looking at COMPAS the algorithm makes it seem that data is inputted by nobody and organisations cannot do anything to mitigate or clean it while the other argument makes it the job of less well paid data entry personnel to input data in ways that foresee eventual data science use cases. Both arguments absolve someone but lead to ignoring the strategic failure by organisations to ensure its different departments work in concert. Regardless if it is a statistical error or a input error organisations ought not to make the error and clearly corporately have abilities to develop better procedures.
I has been hard to assess if COMPAS is still in use the documents remains in use by any user but any google search tends to bring up more academic papers, blogs and the like assessing and criticising its use. I largely hope they got the message.
What is it
The same algorithm is used to evaluate Pretrial Risk Rate to whether a defendant is at risk of absconding before trial. 2 other COMPAS algorithms covered general and violent recidivism the likelihood. I am mostly focused on the violent recidivism as that algorithm could result in a individual serving a more lengthy sentence and being denied parole.
The variables for calculating violent recidivism history of violence, history of non-compliance, vocational/educational problems, the person’s age-at-intake and the person’s age-at-first-arrest. I have found this nowhere else other thank Wikipedia so I am uncertain of its validity.
The given algebraic calculations is below. No i don't know which parameter as it is proprietary and this is not included in any of Northpointe's documentation.
s=a(-w)+a first(-w)+h violence w + v edu w + h nc w
You can see from the algebra how linear it is. If your history of violence and history of compliance was low it would not combine those facts but would be a linear trend. This is contradicted elsewhere with several reporters insisting that the piece of machine learning was a neural network capable of combing values, this may in fact act as a explanation for some that a AI could analyse multiple components from a survey and in combination "guess" a race and therefore become biased against race.
This appears a theme of the media coverage by insisting COMPAS which may have been as simple as the questionnaires you find on Facebook was often reported as being as complicated as the most complex neural network AI. We cannot tell given it's proprietary nature.
In analysing COMPAS ProPublica produced a logistic regression model with some ease that had similar accuracy. Therefore I do not know but assume that COMPAS was much less complex than reported and the media where attempting to exploit fears of AI in order to inflate clicks (though we will never know).
There appears to have been several attempts to find a better model than COMPAS in order to show its flawed nature and estimate what it might have been. The paper The accuracy, fairness, and limits of predicting recidivism (reference at end) crowd sourced 700 suggestions for alternative data combinations both more complex and less complex models showed accuracy equal to COMPAS. Most notably a model with only two parameters age and number of priors was as accurate as COMPAS.
In general most of the alternative models found age a strong predictor and this should be noted as doubly tragic as it would appear likely to sentence younger people whose developmental condition and rest of their lives might be more greatly affected. This raises the question just because a given value predicts a action should we ethically want to?
The group most likely to know what COMPAS was Northpointe routinely uses words like statistics and psychometric in there literature. In there own paper it states that psychometric testing is available upon request FAQ_Document.pdf (northpointeinc.com). . It is possible that some parties are speaking at cross purposes with the media defining COMPAS as a machine algorithm and Northpointe's own guide defining it as psychometric testing. Regardless there remains a perception that COMPAS was a AI and did affect persons sentencing likelihood.
This being said if COMPAS was a psychometric test and not used for sentencing (evidence from court statement indicate it wasn't) then the narrative is vastly changed. A problem here is without open source access to the data and code we will never know. Though this would not resolve the concerns about its bias nor wider concerns about machine learning in court cases.
You could analyse the risk in excel
You can analyse COMPAS inaccuracy in excel. In fact ProPublica have supplied the data for you to do this. Available here compas-analysis/compas-scores-two-years-violent.csv at master · propublica/compas-analysis · GitHub.
The simplicity in the analyst is to convince a reader that simple checks can ensure a organisation is aware of what there tools are doing.
You can see that from the below that African Americans are considered a much higher risk than white people according to Compass. Obviously red is high risk, yellow is medium and green is low. All data is filtered to show where a individual doesn't reoffend.
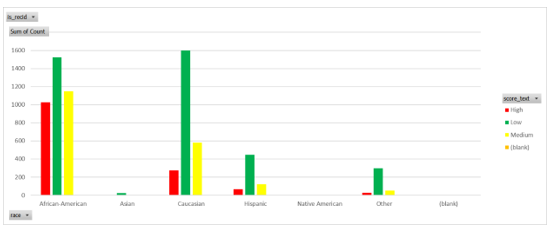
The number gets more extreme when viewed as arrested for violent crime. Not there is no dimension within the data that acts as estimate of the "violence" of the crime. It is just a binary value based on a decision. You probably don't need to turn them into % to describe risk of getting a given category based on race but anyone creating a graph ought to be able to see the risk here. Again risk of being labelled low risk seems to correlate with race but filtered to show where individual didn't reoffend.
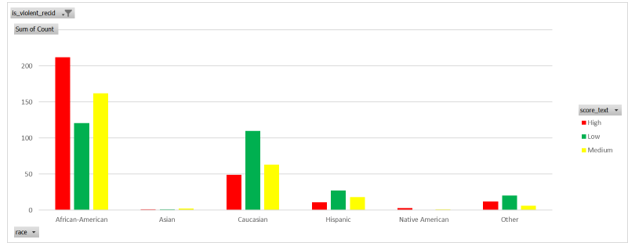
You maybe thinking that maybe X group had more priors and having committed more crimes on average was given a higher risk. The problem with that view is that the average number of prior criminal activity but didn't reoffend. There is a slight difference between groups but not as expected and I am just ignoring that certain racial groups might be victimised by over or under policing so therefore statistically likely to have more priors. Regardless having priors didn't stop a individual from ceasing to reoffend.
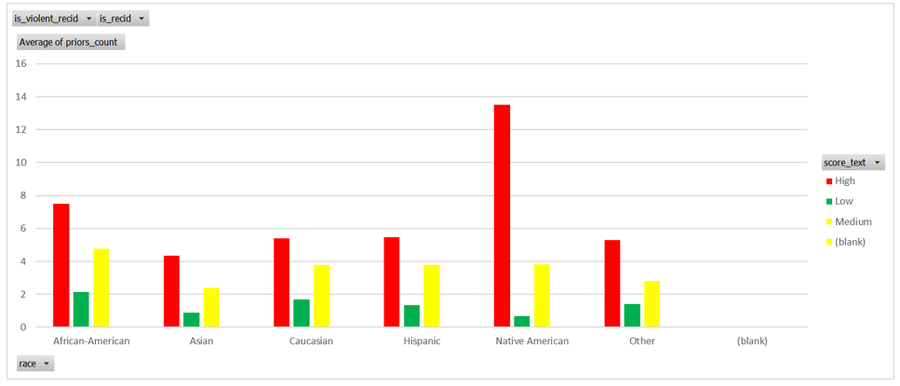
So how about those individuals that do reoffend the below shows the data filtered to show those who do go onto reoffend. It doesn't seem correlated with race and confusingly a high number of those marked green by the algorithm seem to be the highest percentage of those that reoffend.
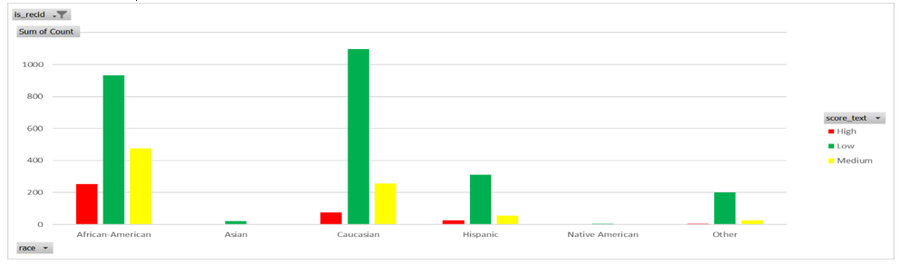
If you look at reoffence rates they do vary with race though it could be argued that they vary with race because of police bias, You could argue that harsh policing is what led to people categorised red to reoffend less. The point is thought you cannot conclude Compass did a good job. You could argue but I am not going to instead I want to suggest the problem here is that systems of justice exist to serve society in assuming that the aim and the discussion of what is the aims of society and the law are a ongoing compact. What is of no small concern is that even if Compas had worked even if it was perfect society would have had to agree the purpose of it's legal systems is to minimise reoffence rates at the cost of all other concerns as the greatest good; say if society decided that maximising only guilty people go to prison or minimise so no innocent people go to jail Compas would be a poor choice of algorithm.
Other algorithms have been used in predictive policing PredPol ended up using police report data to identify areas to deploy more police too. This created a feedback loop of dispatching more police generating more reports meaning more police meaning more reports. Such algorithmic methods are well named as they end up predicting the police themselves not the criminals. And as we can see over policing could cause feedback loops in policing algorithms.
My own view is that you can infer from its data that COMPAS wasn't likely to be a sophisticated artificial intelligence. It goes against my ethics to utilise neural networks to estimate such values but 80% is common ball park value for AI accuracy and 60% seems quite common for linear regression. The first concern here is that estimated accuracy of Machine being 60% might be fine if it affects no-one but when it affects peoples time in prison it should deeply worry us, where it might become biased against peoples protective traits we ought to be deeply concerned as it breaks society values and norms and accuracy seems of lesser concern.
Artificial Intelligence Ethics
The data science community and several corporations have worked hard to create standards for applying artificial intelligence. Let us compare what we know about COMPAS to the standards agreed on by the community.
Data Scientists talk about Human Centred Design this involves posing a number of questions and creating a number of tests and experiments that demonstrate reasoning. The principles are as followed and I try to show how COMPAS failed them and would be unlikely to get consent to be created by a organisation following modern data science methods.
1. Understand Peoples Needs to define the problem: It can be argued that COMPAS the practitioners guide (link below) lists one of the main importance to the legal system being brevity. That is arguably not understanding peoples needs it is understanding what the state wants.
2. Ask if AI adds any benefit to the situation; A Judge still had to make the judgment, a jury had to make a decision and a police officer had to make a arrest. It could not be said COMPAS to have obviated these decisions or automated. If this question was asked understanding of the decision makers of the algorithm would have been made paramount. See next section on what evidence exists on how COMPAS was designed to why I would believe users did not understand the tool.
Questions that could be asked to satisfy this principle are things like is the task boring repetitive and of low value (is a persons freedom of low value?). Or has AI demonstrated above levels of human users (as CORMPAS had reported this was true of average individuals but not about groups and not assessed as compared to specialists.
3. Consider what people could be harmed. There was no evidence I have found that practitioners where asked to routinely test or review whether people could or would bee harmed in its implementation. There is evidence from the Wisconsin Supreme court judgments (discussed later) that Judges where routinely warned how to use COMPAS and how not to be used and specifically stated it ought not to affect chance of incarceration or severity.
That being said more modern takes on data science processes urge remaining on the safe side therefore it seems unlikely if adopted AI ethics that you would conceive as COMPAS as designed was suitable under this principle.
4. Prototype using non AI solutions i.e. build websites that get the feedback and documenting processes right before inserting the AI agent. This ensures that feedback and communication is kept central and not the fanciness of the AI. I have nothing to add here though I note later that evidence is that COMPAS appears from its design documents released to have been more concerned with recreating the decision making of statistics and didn't analyse Judge and law enforcement personnel. The use of the average person on the street as the decision making baseline rather than police or a law related profession as a baseline.
5. Provide ways for people to challenge the system. In COMPAS case the algorithm is used also in parole hearings so the chances of getting a rehearing or challenge was also diminished. Furthermore COMPAS clearly being a proprietary algorithm frustrated ability to challenge. It is clear that concerns about intellectual ownership was put ahead of reputational damage; that didn't appear to have worked out well for them.
6. Build in measures to demonstrate to users how people are actually using it as they are using it. As we have seen from the above a simple review in Excel would potentially have allowed a user to raise some concerns. I have not been able to see any evidence that additional dashboarding and analytics where supplier. You can see from that the previous excel analysis that if this was done it could actually be quite simple to analyse COMPAS if the data was readily available.
A important paper that i have seen cited in several books has been A Framework for Understanding Sources of Harm throughout the Machine Learning Life Cycle by HARINI SURESH and JOHN GUTTAG. The link is available here A Framework for Understanding Sources of Harm throughout the Machine Learning Life Cycle (arxiv.org).
The paper attempted to standardise language focusing on sources of harm within ML. The paper identifies 7 main sources of harm that have to be evaluated or reduced in developing a model. I recommend reading the paper directly I merely intend to talk about them briefly in light of COMPAS.
1. Historic Bias: The data is complete and ordered but data sets accrue over time and may represent how it was done "back then" and not represent the current reality. It is possible COMPAS was compromised by the historic data and it must be noted that it was not centuries ago that certain forces within American politics where more prominent.
2. Representation Bias: This happens when certain groups of people are under or over represented in the data. I have not found anything though reviewing the practitioners guide there is little evidence that the model was directly assessed against real contexts but was compared to other known models quite frequently. Therefore it would be hard to assess representation this is directly called Comparison bias below and has its own category but I discuss here to indicate I found no solid figure of population used to build the model there was a number of Northpointe's own documents i had not read having read the practitioners guide and thought it terrible,.
I did later find another document from Northpointe which said that COMPAS has several different Norm groups and then listed them. Though decided this was not the same as demonstrating removal of representational biased and was somewhat concerned as it wasn't clear how the same algorithm could be optimised across every group in every situation and suspected that might be another form of bias.
3. Measurement bias: This is where some value encodes a meaning within the dataset that improperly represents the intended target. I.e. in the case of believing that race was derived from postcode/zipcode and therefore was improperly represented in the model.
4. Aggregation Bias: Is the belief that some variable has been aggregated wrong. A example in this instance might be race itself. Evidence from police database records showed race itself may have been improperly applied and regardless multiple cultures and sub cultures can end up lumped under certain grouping that maybe ignores the sub differences. It is worth considering it is basically impossible from the data the police had to identify mixed race individuals in their own data.
Given that the likely proxy for race within COMPAS data set was postcode/zipcode it is clear that COMPAS had very little likelihood of not including aggregation bias. Though it should be recognised postcode in this sense was being used by COMPAS/Northpointe to represent social economic background and not race; it just appears a unfortunate truth that it correlates with race either as much or better and may have resulted in a feedback loop if data about the arrests, trials and parole hearings was used to reinforce COMPAS (and we may as well assume it did happen over the 15 years was in operation).
Another example was it is reported by ProPublica used FBI definition of violent crime and defined priors as only previous convictions and recidivism as arrest and conviction. Propublica has suggested these definitions may not have been the definitions used by Northpointe and could have led to more bias during deployment (see below) where local law enforcement arrived at different definitions (an example how one type of bias can compound another).
5. Learning Bias; describes how bias can arise depending on the way the AI learns because the function is mismatched. Upon reviewing the COMPAS practitioners guide it mentions that the 1-10 assessment it produces correlates to a % score chance of recidivism. Though instead of trying to express values natively as percentages a arbitrary 1-10 value was chosen.
The potential learning bias of COMPAS can also be interpreted as Aggregation and Deployment bias (see below) as in practice and as seen in the excel work above the 1-10 score was often represented to decision makers as a Low, Medium or High risk category rather than a number.
6. Comparison Bias: This is the bias that arises from comparing models to other models and not to testable live re world decisions. COMPAS own documentation does this on a scale I found hard to stomach in fact I struggled to tell if they had ever tested it entirely and directly on real world outcomes as every paragraph referenced other groups test data.
7. Deployment Bias: This is bias that arises because there is a difference between how it was designed and how the model is used in reality. There seems a suspicion that COMPAS was affected by this but it is hard to quantify it was in such widespread use that fragmentation in use must have occurred. Likewise I didn't recognise within the COMPAS practitioner guide a effective, simple and well documented user guide for practitioners.
In conclusion I would conclude that the framework would have helped avoid and assist in developing COMPAS better many of its failing fall neatly into its structured view of how harm manifests in Machine Learning and Data Science projects.
Another paper that has been instrumental in Data Science circles is the four fairness criteria. The language comes from On Formalizing Fairness in Prediction with Machine Learning by Pratik Gajane 1 Mykola Pechenizkiy the paper is available here On Formalizing Fairness in Prediction with Machine Learning (arxiv.org).
And in comparing the formulations we can see why both Northpointe and ProPublica can make contradictory claims that COMPAS is fair or unfair and both arise due to different formulations about being Fair. The four fairness's arise because we can discuss Parity Versus Impact and Treatment versus Preference.
I have not found the direct statement made by Northpointe but Northpointes argument appears to be both white and black overall had similar recidivism rates. I try to fit morale arguments to the four types of fairness discussed in Pratik Gajane 1 Mykola Pechenizkiy paper.
1. Parity/Treatment: A Algorithm can be fair because it treats people roughly the same. Northpointe may argue that black people and white people have a parity of recidivism and are treated and processed in the same way it is fair. This seems to be the view taken by COMPAS supporters.
2. Parity/Preference: A Algorithm can be fair because it treats people roughly the same. Northpointe may argue that black people and white people have a parity of recidivism and we attempt to accommodate their preferences and deal with complaints it can be fair. In this view we may process data using COMPAS as it has parity but i must consider a users complaints over where they have been mistreated. This seems a position similar taken by Wisconsin Supreme court (see below) that while the algorithm may have specific instances every chance to rectify and analyse the data is given to both sides.
3. Impact/Treatment: We ought not to have differences of Impact across race or other protected the conclusion taken by Propublica appears upon these lines. The algorithm is unfair it impacts people differently and they get different treatment.
4. Impact/Preference: In practice this means fairness by unawareness we realise people can be impacted and foreseeing a preference to not and likewise desiring not to remove this data from the system. There is every reason to believe from Northpointe statements that race was not included in the data and elsewhere we have discussed the impact of proxies affecting the data.
Therefore while it has been quite common for the media to discuss COMPAS in disparaging concerns (and sometimes I have failed to help myself) there are logical arguments that can be made that COMPAS was "fair". I personally assess it as a unfair algorithm but realise there are potentials others will formulate the argument differently.
Though even with a difference of opinion it is fair and reasonable to Ethically contest that COMPAS ought not to be used still based purely on the maths. COMPAS and Northpointe's defence that overall COMPAS gives same assessment regardless of race and ProPublica assessment it was unfair given that it tended towards false positives for one race and false negatives for another can be assessed as wrong if we consider we are more concerned with the number of innocent people a system of decision making sends to jail than any other metric. To do this shows why AI ethics might be highly important for organisations in the future as there appears to be a risk that we build the AI that reflect our values and testing not the AI that we think we will make in the lab.
To do so would require discussing the risks of False Positives and False Negatives and in light how it might affect different groups. We also might attempt to ensure that such groups are contacted and canvased ahead of time to identify any future issues.
For this reason its should be obvious that any AI project should analyse and risk assess against impacts to any stakeholders and generate a range of graphs like the ones shown in my excel work.
How Was COMPAS Designed
The practitioners guide to COMPAS can be found here FieldGuide2_081412.pdf (northpointeinc.com). The user guide for COMPAS says the following.
COMPAS is a fourth generation risk and needs assessment instrument. It is a web-based tool designed to assess offenders’ criminogenic needs and risk of recidivism. Criminal justice agencies across the nation use COMPAS to inform decisions regarding the placement, supervision and case management of offenders. Empirically developed, COMPAS focuses on predictors known to affect recidivism. It includes dynamic risk factors in its prediction of recidivism, and it provides information on a variety of well validated risk and needs factors designed to aid in correctional intervention to decrease the likelihood that offenders will re-offend. COMPAS has two primary risk models: General Recidivism Risk and Violent Recidivism Risk. COMPAS has scales that measure both dynamic risk (criminogenic factors) and static risk (historical factors).
Statistically based risk/needs assessments have become accepted as established and valid methods for organizing much of the critical information relevant for managing offenders in correctional settings (Quinsey, Harris, Rice, & Cormier, 1998). Many research studies have concluded that objective statistical assessments are, in fact, superior to human judgment (Grove, Zald, Lebow, Snitz, & Nelson, 2000; Swets, Dawes, & Monahan, 2000).COMPAS is a statistically based risk assessment specifically developed to assess many of the key risk and needs factors in adult correctional populations and to provide decision-support information regarding placement of offenders in the community. It aims to achieve these goals by providing valid measurement and succinct organization of many of the salient and relevant risk/need dimensions. Northpointe recognizes the reality of case management considerations and supports the use of professional judgment in concert with actuarial risk/needs assessment. Following assessment, a further goal is to help practitioners with case plan development/implementation and overall case management support. (SIC)
That was a lot of PHD level quotations and not much substance. The Company go onto describe Compas as useful across the inmates lifecycle.
COMPAS Core was developed to be used with criminal justice clients at any point during their supervision and can be used as a reassessment tool. The Case Supervision Review screening (23 items) has been built into all COMPAS sites as an optional method for periodic review of the person’s status and progress.
From the field guide other issues concern me, a score 0f 4 means "he" (sic) has 60% greater risk of reoffending than a regular person. The output equates to a percentage but instead we are given a arbitrary value measured in increments of 4. This obfuscates actually percentages behind arbittary numbers. By the way the Compas score was calculated as a 1-10 so the above doesn't make sense as if a 1 is zero or average then 5 is 50% and a 9 is a 120% chance of reoffending (how do you get over 100%). This is highlighted to show when attempting to put Compas in simple terms one finds lots of inconsistencies.
Section 2.4 moves onto Criminal Theories what happened to the levels? There are 5 theories it isn't stated which one COMPAS is based on, none are justified with data. It discusses the AIPE model so there is a COMPAS score of 4, levels, a Criminal Theory, and now the AIPE I'm on page 11 of 62. Oh great section 2.5 splits scales into basic and risk scales and raw scales into decile scores which is a table and yet still none of this is raw statistical data it keeps referencing the norm group and then says a low decile score does not indicate a low risk of violence (really i thought that was the point was to measure inmate reoffence risk).
Another guide was somewhat better FAQ_Document.pdf (northpointeinc.com). This appears better written and while doesn't include any clues to COMPAS design seems a later version being from 2012 and aimed less at the PHD level specialists. It includes reference to inclusion of a validity test to flag potentially dishonest or misleading answers by counterfactually including other answers. Again despite COMPAS secretive nature this is a technique for social scientists producing psychometric tests and not data science. The fact that guidance was updated over time might also indicate that he algorithm itself changed over time making it even harder to consider its development. Though likewise one could see why Northpointe wanted to retain COMPAS proprietary status trainers fees start at between $1200-$1300 a day!
I realise I am being very disparaging of COMPAS in reviewing this aspect of its documents. Though my opinion is it is overly technical without being mathematical grounded and inappropriate for level of practitioners that are the target audience. It also seems to rely on a litany of referencing other literature rather than demonstrating its own testing methodology. I feel that this shows that we ought to insist in much more simplistic language, ethics, and governance. I say this because Machine Learning can be made explainable.
Machine Learning Explainability
Everything we have discussed assumes that any piece of machine learning is by its very nature a black box. We have somewhat put that under doubt by investigating the model using excel though surely there ought to be better tools for data scientists. A lot of this I learned from Kaggle; though nowadays several comparable courses exist find it here Learn Machine Learning Explainability Tutorials | Kaggle.
Alternatively it could have been managed by doing hypothesis testing to see what variables covaried with COMPAS and these values that covaried could be investigated. If Northpointe had spotted postcode varied with errors, or another metric you might consider investigating. And this gives the biggest criticism of COMPAS there are methods available from Data Science for investigating and establishing a models accuracy and assessing roughly how it is arriving at its decisions.
While the aggregate model is complex it can be rationalised down to a series of graphs and plots and varied metrics. These varied metrics can be used t produce graphs and can be done to provide explainability to even sophisticated AI models as they rely on scrambling given parameters and seeing if the change positively or negatively impacts the models performance. Regardless of if new techniques are available hypothesis testing has been a available technique for centuries.
Partial dependence plots, Shapley Values and Permutation Importance where all developed during the time COMPAS was in use (though after the reported 1999 beginning of development). The 1999 creation day might mean we should give Northpointe the benefit of the doubt it can be argued to be dated and old technology and the media interest that emphasised it being a neural network or AI might be concluded to be unlikely. It appears to be a statistical formula and maybe over the decades its environment changed, likewise new technologies have emerged.
Though has society been changed by COMPAS? Is it now unthinkable for another COMPAS to be made?
Legal impacts
The court case appears badly reported upon in the media. The media seem to have tended to report several times i found statements by media reports that contradict court documents. Conversely I am no Lawyer i recommend reading original sources in each case and considering for yourself the below is mainly a summary.
The lower court made the sentence on the basis the defendant was found driving a car used in a shooting as well as tried to elude the arresting officer. The pieces of evidence that led to the conviction was this and the estimate by COMPAS that concluded the defendant was at risk of reoffence.
The key issues appear to be the court refused to share the COMPAS document a right the document admits to be at the courts discretion a decision that appears agreed to be erroneous by the supreme court. This can be compared to many assertions by media that focused on sensationalising the AI aspect.
On appeal in State v Loomis was not heard by Wisconsin High Court and by doing so they affirmed the use of COMPAS as evidence by a lower court. The contestation was that race and gender had been included in COMPAS data and as discussed in this article there appears no evidence stated by a authority that says this is the case and so it is possible the case was dismissed on grounds that it was not competent.
A copy of the decision can be found here STATE v. LOOMIS | FindLaw. In it the court alleges that the drive by shooting was not the basis of the criminal arrest but the inputting of a guilty plea to other crimes including evading a police officer. According to papers does not appear agreement that COMPAS directly led to the incarceration or the severity of charges nor the length.
It is mentioned in the statement by the court that originally COMPAS had only been used by smaller number of practitioners at the urging of the American bar association.
Harvard review reported that the Court had stated that the court had made statement that mentioned Geneder was included but only for the purpose of accuracy. Harvard review also stated that the court had ruled a requirement to ensure confirmation of data and accuracy of a model being used State v. Loomis - Harvard Law Review . The court appeared mostly satisfied that the defendant had been shown data but not the PSI (output document) and was more concerned that defendants be given every chance to review and analyse the data used and potentially the model at trial.
In making this statement the court agreed with Loomis ought to as being the person best set to correct any mistakes in the data have full access to the data and understanding of the algorithm. Though didn't agree that the lower court had formed its opinion solely based on COMPAS nor had used it to arrive at a worse punishment.
The courts criticism of COMPAS focused on its secretive nature, its workings where not properly understood by the judge, the defence or the court at large due to its proprietary nature. The court stated COMPAS and similar algorithms cannot be used “to determine whether an offender is incarcerated” or “to determine the severity of the sentence.”
Harvard review also states that the Judges using COMPAS receive 5 written warnings. When taking together these 5 warnings not to use it. From my review of COMPAS above I personally suspect COMPAS was a flawed model that appears to fail basic tests of bias. This being said i am satisfied that what reported in the media as AI being used to sentence people based on race.
Anne L Washington has argued that new standards of data science has to be developed to argue with algorithms. I personally prefer the idea that algorithms may inform police investigation but standards of evidence and principles of Jury led sentencing ought to be jury led. It does not feel terrible if a AI helps a police officer find evidence that sentences the guilty it appears another proposition if a algorithm advises a judge to send a innocent person to go to jail.
This has been a illuminating read In State v Loomis, COMPAS was the subject of a legal court case where a Judge sentenced a man to two years imprisonment based primarily on the COMPAS score predicted a reoffence. This conviction was upheld under the interpretation by the court that the algorithm must be faulty due to improper and dirty data and the defendant had access to both data and prediction and could have corrected it. In this article Anne L Washington argues that the court failed to consider that the algorithm itself could have been faulty or improperly designed and urged the need for ability to challenge the quality of the algorithm itself using data science techniques.
This should be seen as important as being proprietary the inner workings and specifics of the Algorithm remain unknown. This should be concerning as the use of code utilising generally understood mathematical principles seems unlikely to be awarded a protected patent so it does not seem to affect the rights of Corporations directly to require open discussions on there algorithms. Likewise AI development is filled with specialised AI and so a patent or otherwise sensitive intellectual property being a part of a given algorithm appears possible.
I would hope in future if provided the explanation of the model, its output, a chance to review the inputs as well as better standards for data science evidence in courts argued by Anne L Washington that courts and governments can do better. While it is insisted upon by the courts that COMPAS did not change the decision of the court there is a problem that in this specific case the algorithm could not be put on trial.
Algocracy
Algocracy means rule or governance by algorithms.
There has been over time a proliferation of legal expert systems as law is a expansive and difficult subject an approach has been to use more AI or search algorithms to automate decisions and gather information. Therefore we increasingly move towards Algocracy or governance by algorithm as increasingly so many objects within life will be administrated by machines; if not in full then at least in part.
I feel that COMPAS here neatly demonstrates the pitfalls of such a approach and how the court did not stop its use but rather required greater sharing and transparency of data. I also feel there maybe a deficit here in that the court has not expressly stated a right and or a process to review and challenge the algorithm itself only implied its necessity.
It is concerning that a machine may advise someone to send you to jail and how long to keep in you in jail. Though the Judge who took its advice is told essentially not to take its advise for any meaningful decision, though the machine itself can not be taken to court. It does not appear that anyone is responsible for the decisions made by COMPAS which it has been ignored that if it has little no affect on Judges decisions should be logically be stopped as a waste of money.
Alternatively if these algorithms do mean something and do have a impact then it is plainly required and in the public interest to be aware of there use and have rights to challenge these tools just as any other evidence or accusation can be cross analysed in court.
It is plainly true not everything said about COMPAS was true by all parties; though this did not stop the media and reputational damage that happened. Likewise there seems every reason to suspect that data science has been lumped in with psychometric analytics and there is a problem if because of proprietary nature that innocent people are affected by desire to automate we may wish to forego the automation.
COMPAS to me is a great example of a bad project. It in my mind will be the anti pattern of what i think data science ought to be and while it is harder to drawn conclusions from a anti pattern to what one ought to do as a positive exhortation towards best practice I offer the below as my lingering questions.
Data ought to be reconsidered as a important strategic asset and its use ought to be reviewed across the organisation. All the statements made across the media seem to focus and glamorise one specific aspect and ignore that most of these issues are issues that larger organisations can resolve. The focus on discussing police bias or machine learning bias miss there ought to be some function that manages these assets as data is input somewhere and if the machine learning used has value it also needs to be actively managed. This appeared to be missing. Further to this COMPAS was not new and bleeding edge tech and such more active management might have adopted a better replacement that was clearly available.
Analysts with excel knowledge if asked to question basic data assumptions could detect the issues around COMPAS easily enough. Full data scientists could and should create code through machine learning explainability that by running can show the short comings quickly. This can be contrasted with COMPAS practitioner guide which excessively quoted scientific papers and was not testable being proprietary. It might be too extreme to argue that therefore proprietary machine learning is problematic but it at least should be used as the basis to require some level of understanding of what is being bought.
Data scientists should be held to project and ethically standards using AI ethics which should be part of good corporate governance. Finally like the courts legal decision supported customers should be able to know and challenge how there data can be used and given chances to correct though also like the courts a mistake ought not to be instantly assumed to mean that every other conclusion is invalidated as long as we can on review justify reasoning.
The reason all this additional project and corporate governance should be that part of the reason that Wisconsin supreme court rejected the appeal seems to be the 5 warnings that COMPAS had as part as its policy document. For all the media's insistence that COMPAS was a AI used in sentencing the courts policy documents insisting otherwise was a good start.
My final concern and really the only solid conclusion I came away with upon researching COMPAS was that we appear to be heading for rule by Algorithms the need to keep up with the increasing amount of work generated by normal life. COMPAS seems obviously flawed it is concerning that there is the potential that not only might certain facets of life be managed by algorithms in the future but they firstly might be flawed models or otherwise no one might be consulted about there implementation one day decisions taken by judges might be replaced by machines.
Though rather than state this as the problem it is also clear that data science has the tools within AI ethics and machine learning explainability to resolve it's own problems and explain its own short comings. The story unlike the media reporting is not necessarily we need to avoid use of data but instead we might need more data science to guard against the abuses of data science in decision making.
References
Dressel J, Farid H. The accuracy, fairness, and limits of predicting recidivism. Sci Adv. 2018 Jan 17;4(1):eaao5580. doi: 10.1126/sciadv.aao5580. PMID: 29376122; PMCID: PMC5777393.
Otherwise all material was attained through open sources and its use is consentient here with fair use for criticism and evaluation.
Add comment
Comments